Business Viability Index: AI support for business planning and avoiding failures
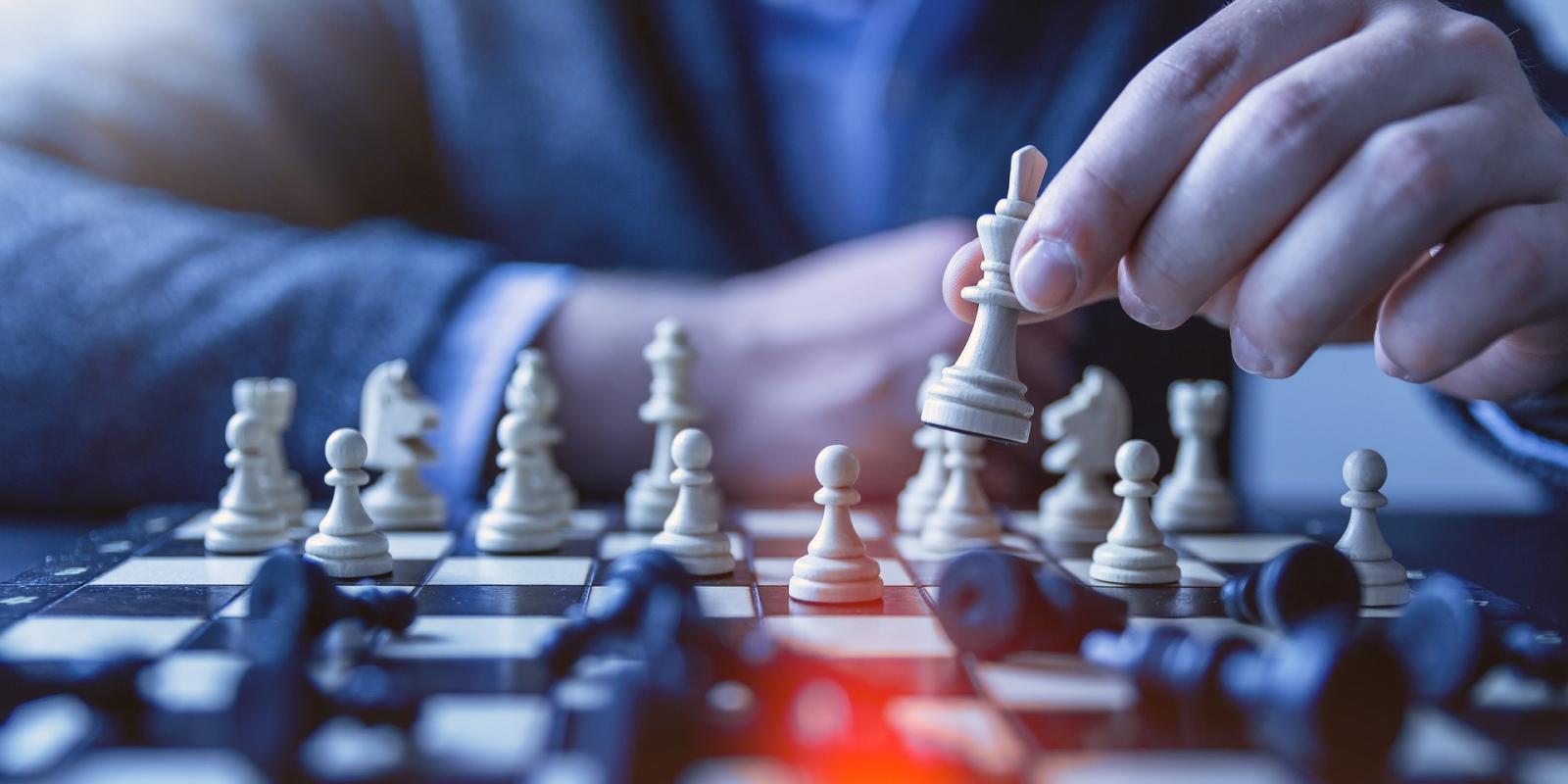
The proposed solution leverages the AI concepts to analyze market and business operational data in real-time and derive actionable business management fundamentals in the same timeframe as data is analyzed.
Machine learning, supervised learning and unsupervised learning concepts are used along with deep learning neural network solutions to learn from market and business behavior patterns and use the derived knowledge to support management decision-making process. The essence is to incorporate a huge amount of available data and leverage the interrelationships between different data sources to derive a summary actionable basis for real-time decision making.
[June 2023] The solution is currently a work in progress.
Tactical Objectives
The planned AI business support system is designed to achieve the following tactical objectives:
- creation of an analytical report on the respective business activities and market conditions;
- if relevant, generation of risk scenario-based alerts to review and change the course of business action;
- if relevant, production of performance recognition notes to emphasize whether the business is market oriented and successfully run;
- generation of solutions to address the risk scenarios when identified;
- forecasting the outcomes of the solutions offered;
- allowing business representatives to enter business-specific data into the system and to create the forecasts based on these additional inputs.
Methods
The proposed solution uses machine learning and artificial neural network tools to ensure the achievement of tactical objectives. From a technical point of view the system is able to perform the following activities:
- it learns the patterns of business practices within the given business sector;
- it identifies site-specific issues impacting operations at a particular geographic location;
- it learns the business practices of a particular business entity;
- it evaluates the business practices of certain companies on industry and location-specific issues;
- it determines the need for communicating specific risks;
- using the available data inputs and considering potential customer additions, the system forecasts the possible development scenarios for the respective business unit, including corporate finance analytics as well as concepts such as net present value, weighted average cost of capital, internal rate of return, return on investments, customer acquisition costs, breakeven analysis etc.;
- it communicates generated development scenarios as risk mitigation strategies or reinforcements of positive outcomes.
Functional requirements
The solution is designed to be user-friendly, requiring no specialized competencies. Intuitive menu systems facilitate easy navigation. Operational costs are minimal unless substantial data updates or securization are required.
Customization costs and time depend on the extent and essence of customization needed. In case of replacement of data sources from one “similar” data to another, the customization is efficient and the respective code is provided to users. The same applies if some data sources need to be excluded (not all data may be available in similar format in all regions).
Results and impact assessment
By active usage of this AI support system, it is projected that the bankruptcy rate will decrease by 10% within three years, while overall insolvency will improve by 25% during the same timeframe. These positive impacts on business viability will contribute to enhanced economic stability.
Dependencies and constraints
The implementation of the Business Viability Index AI support system is subject to the following dependencies and constraints:
- Reusability and minimal dependencies: the solution should be designed to maximize reusability and minimize reliance on specific software solutions, promoting flexibility and integration with existing systems.
- Scalability and integrability: the system should be scalable to handle increasing data volumes and integrate with other relevant systems within the organization.
- Data availability and interoperability: the system should utilize diverse data sources and adapt to changes in data formats or sources, ensuring compatibility and effective data integration.
- Documentation for interoperability: comprehensive documentation should be provided to facilitate seamless integration with other systems, enabling proper configuration and connectivity.
- Access control and data privacy: the system's output should be protected and accessible only to authorized personnel, complying with data privacy regulations and safeguarding sensitive information.
The risks of the solution are wrong and misleading evaluations to particular companies’ operational statuses, misleading forecasts produced and inappropriate development scenarios communicated. These risks have been mitigated via extensive testing of the solution and evaluation of the results received from testing. Experts and industry professionals are being involved in evaluation process judging AI technology trustworthiness.