AI-driven fraud detection for grant family allowances (Groeipakket)
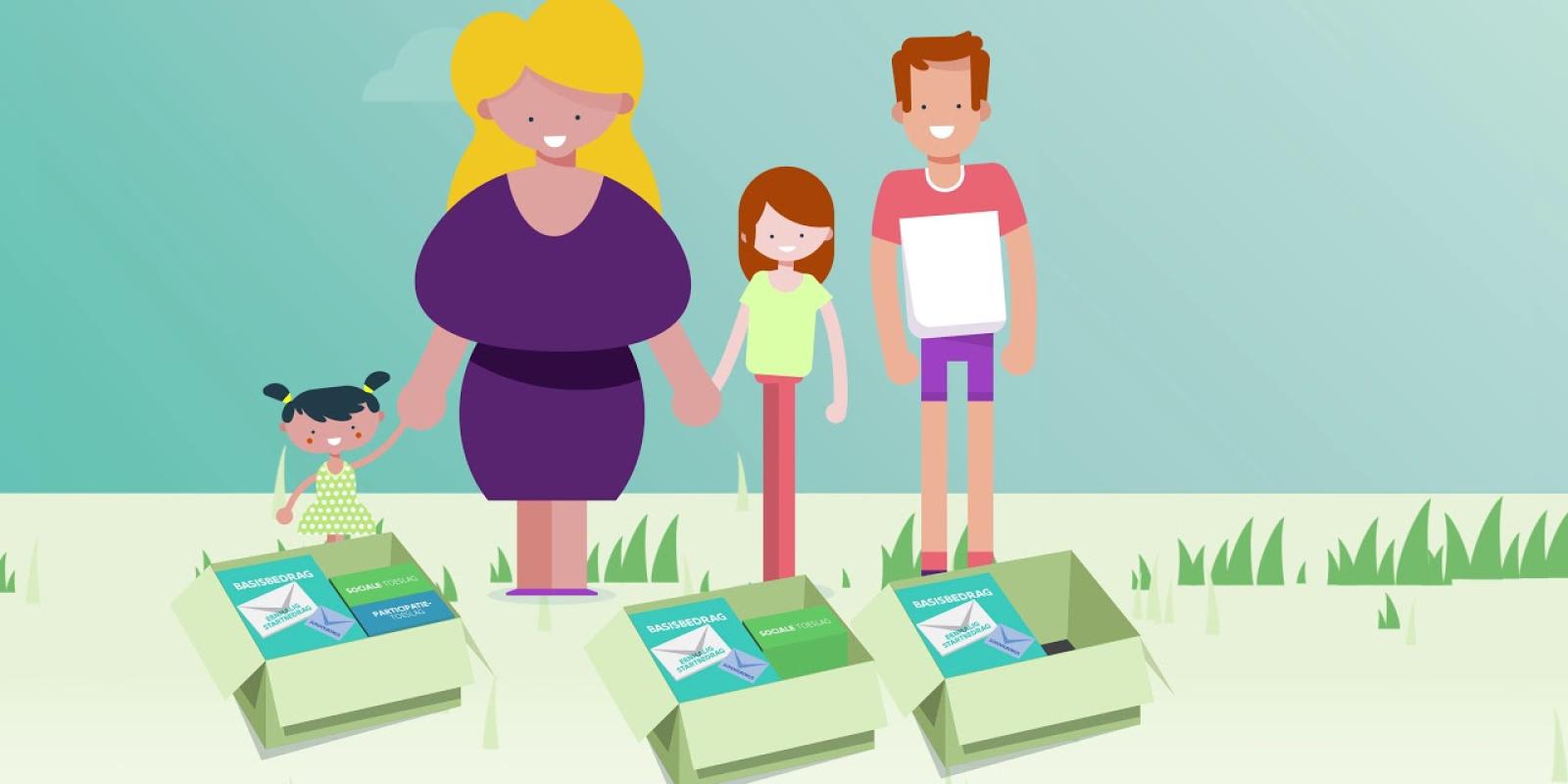
Abstract
An AI-driven fraud detection system is to provide insight into files with an increased risk of fraud and improves the work process of file managers and social inspectors by making risk-oriented choices. The fraud detection by this solution is based on elements that were identified in past fraud cases. When the solution has identified a possible fraud case, the inspector confirms the fraud case. Humans and products work in a reinforcing manner this way.
Tactical Objectives
- Providing insight into files with an increased risk of fraud
- Improving the work process of file managers and social inspectors by making risk-oriented choices
Methods
The development of the fraud detection system will be accomplished in 3 phases:
The first phase is the preparation phase in which some preliminary studies were done by the company SAS. Also some interviews were taken to get an idea of problems in the public sector that could be tackled by AI.
The second phase was the exploratory phase. This takes the longest time of the 3 steps.
During the first step, we try to gain as many insights, ideas and inspiration as possible during the ideation workshops. These ideas were then transformed into different use case templates to which a prioritization matrix was attached to see if the case is prioritized to other projects. The goal here is to develop a use case from an idea that the end users can impact positively. This was achieved by organizing workshops, inspirational sessions and creating use case catalogs. The workshops were divided into 3 different themes: people & individuals, internal operations and environment & mobility. During the workshops, 4 principles were central, being the use case driver approach, the inspiration and usability of different building blocks in other designs, co-creation & collaboration and agile development.
A second step within the exploratory phase is to organise a design sprint, followed by the final step the development of the prototype.
The third stage is to develop a business case. This phase has not yet started. The phase we are in now is the development of the prototype.
Functional requirements
The idea for this solution was the result of an internal call AI challenge. The “Agentschap Uitbetaling Groeipakket (the agency responsible for the allocation) Growth Packages came up with the idea of an AI-driven fraud detection tool. The prototyping of this solution was facilitated by an external party ML6. During the design sprints, the agency and ML6 looked closely together at how the solution would fit into their daily operations, ethical implementation and explainability of the prototype.
Results and Impact Assessment
The fraud detection application was still in a prototype phase while writing this text.
However, the results of the design sprints show us that data sources are reliable for prototyping, that there is explainable AI that clarifies why something is flagged as fraud and that there are possibilities of interaction between humans and AI while it does not create extra workload. It supports the core task of the inspection team and fits the current way of working.
Dependencies and constraints
- Identification of the files is still to be examined and would increase the quality of the selection; using a classification algorithm
- Gathering new insights through comparison with most similar files in the past is needed to make sure that the fraud detection tool is making correct fraud claims